Top 5 Trends in Deep Learning to Watch for Aspiring AI Enthusiasts
Introduction to Deep Learning Trends
As the field of artificial intelligence continues to evolve, deep learning remains at the forefront of technological innovation. For aspiring AI enthusiasts, staying updated with the latest trends in deep learning is crucial. Here, we explore five key trends that are shaping the future of this dynamic field.
1. Transfer Learning: A Game Changer
Transfer learning is revolutionizing how we approach deep learning models. This technique allows models trained on large datasets to be repurposed for tasks with smaller datasets, saving time and computational resources. By leveraging pre-trained models, developers can achieve high accuracy with less data and effort.
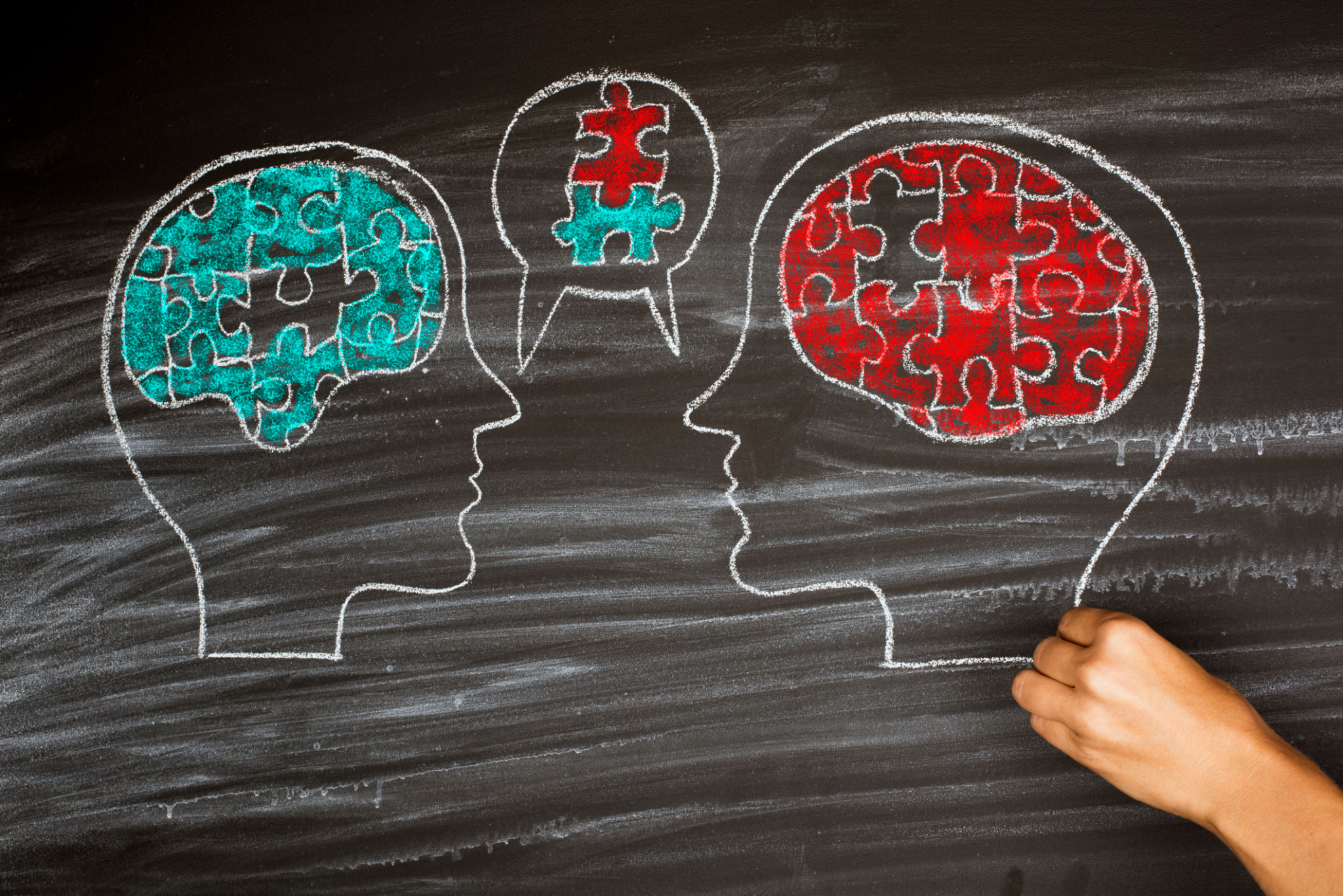
This trend is particularly beneficial for industries where data collection is challenging. For instance, in healthcare, where patient data may be limited or sensitive, transfer learning enables effective model training without extensive data gathering.
2. Explainable AI: Understanding the Black Box
As AI systems are increasingly integrated into critical decision-making processes, the need for explainable AI (XAI) has become paramount. XAI aims to make AI models more transparent and understandable to humans, addressing the "black box" nature of deep learning models.
Methods such as attention mechanisms and feature visualization are enhancing our ability to interpret complex models. This trend is vital for building trust and ensuring ethical AI deployment, particularly in sectors like finance and healthcare.

3. Federated Learning: Privacy-Preserving Collaboration
Federated learning is transforming how we approach collaborative model training. By allowing multiple devices or organizations to train a shared model without exchanging raw data, this technique enhances privacy and security.
This trend is especially relevant in areas where data privacy is a concern, such as personal devices or cross-institutional collaborations. Federated learning enables the creation of robust models while keeping sensitive information decentralized and secure.
4. AutoML: Democratizing AI Development
AutoML is simplifying the development of AI models by automating the design and optimization of machine learning workflows. This trend empowers individuals with limited expertise to create effective models, accelerating AI adoption across various industries.
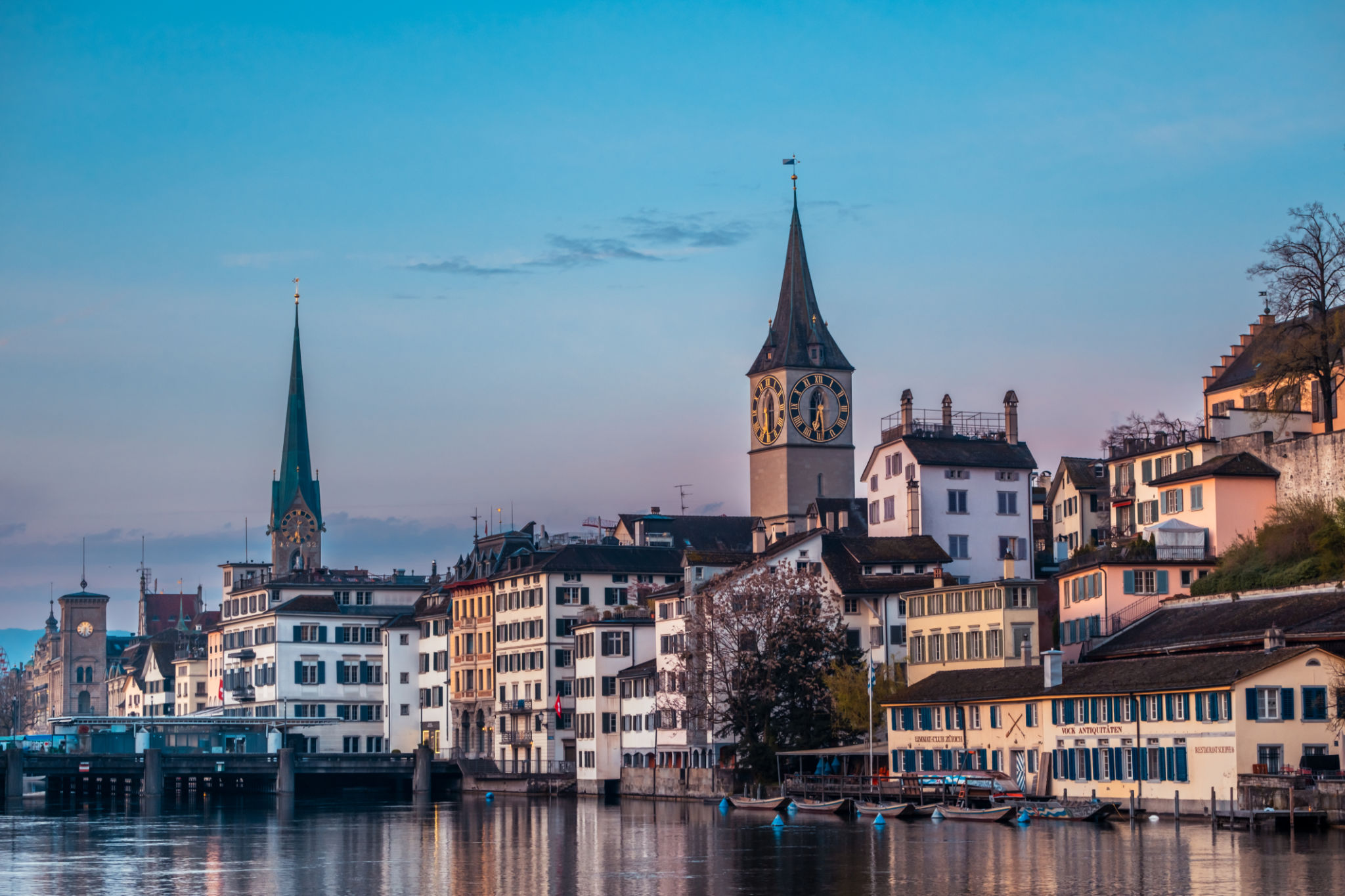
By streamlining processes such as hyperparameter tuning and feature engineering, AutoML reduces the technical barriers to entry, making AI accessible to a broader audience.
5. Edge AI: Bringing Intelligence Closer
Edge AI involves deploying AI models directly on devices rather than relying solely on cloud-based solutions. This trend enhances real-time data processing and reduces latency, which is crucial for applications like autonomous vehicles and IoT devices.
The advancement of hardware technologies and model compression techniques is driving this trend, making it possible to run complex deep learning models efficiently on edge devices.

Conclusion
The landscape of deep learning is rapidly evolving, with these five trends paving the way for future innovations. For aspiring AI enthusiasts, staying informed about these developments is essential for navigating the exciting world of artificial intelligence. Whether it's through transfer learning or edge AI, embracing these trends will shape the future of technology as we know it.