Common Misconceptions About Machine Learning: Debunked
Understanding Machine Learning
Machine learning has become a buzzword in today's tech-driven world, yet many misconceptions persist about its capabilities and limitations. Understanding what machine learning truly entails can help dispel these myths and provide a clearer picture of its potential. In this post, we will address and debunk some of the most common misconceptions about machine learning.

Machine Learning is the Same as Artificial Intelligence
A frequent misconception is that machine learning is synonymous with artificial intelligence (AI). While they are related, they are not the same. AI is a broader concept that involves creating systems capable of performing tasks that would normally require human intelligence. Machine learning, on the other hand, is a subset of AI that focuses on the development of algorithms allowing computers to learn from and make predictions based on data.
Machine Learning Can Work Without Human Intervention
Another myth is that machine learning systems can function entirely autonomously without human involvement. While it's true that machine learning algorithms can process and analyze data independently, humans play a crucial role in setting objectives, selecting the right datasets, and interpreting results. Human oversight is essential to ensure these systems operate as intended and align with ethical standards.

Machine Learning Always Provides Accurate Results
It's easy to assume that machine learning algorithms are infallible, given their complex nature. However, this is far from the truth. The accuracy of a machine learning model depends on several factors, including the quality of the data it is trained on. Poor-quality or biased data can lead to inaccurate predictions. Continuous monitoring and updating of models are necessary to maintain their accuracy over time.
The Complexity and Limitations of Machine Learning
Machine learning is often perceived as a magic solution capable of solving any problem. While it offers impressive capabilities, it also has its limitations. Understanding these limitations is key to setting realistic expectations for what machine learning can achieve.

More Data Always Leads to Better Models
While it's true that machine learning models require large datasets for training, more data doesn't automatically mean better results. The quality of data is more important than quantity. Datasets must be diverse and representative of the problem being solved. Additionally, excessive data can sometimes lead to overfitting, where the model learns noise rather than useful patterns.
Machine Learning is Only for Tech Giants
Another common misconception is that only large corporations with significant resources can benefit from machine learning. In reality, machine learning is accessible to businesses of all sizes. With the rise of cloud-based platforms and open-source tools, smaller companies can leverage machine learning to gain insights and improve their operations without needing extensive infrastructure or expertise.
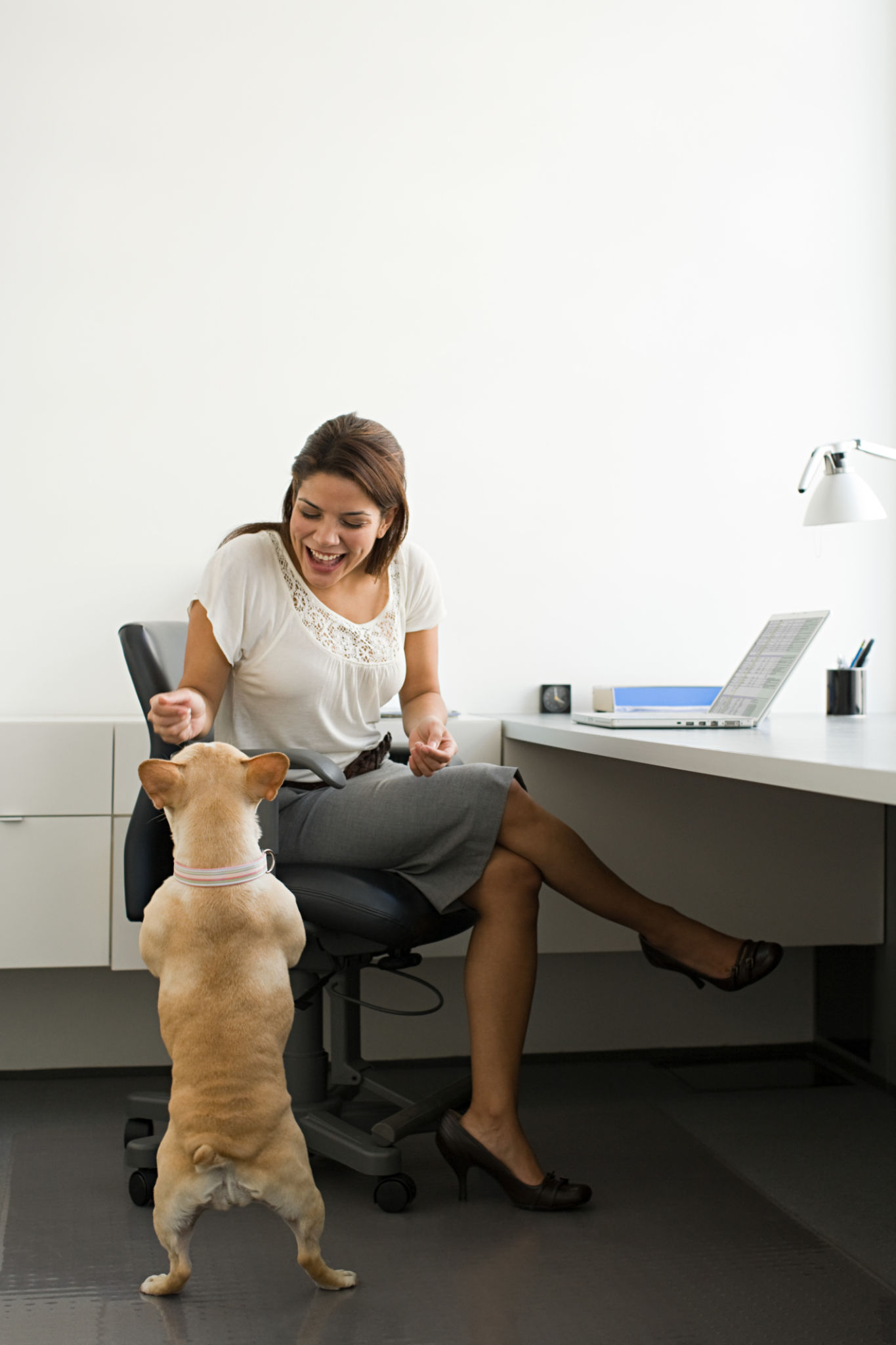
Conclusion
By debunking these misconceptions, we hope to provide a clearer understanding of what machine learning truly entails and its potential applications. As technology continues to evolve, having a realistic perspective on machine learning will enable individuals and organizations to harness its capabilities effectively and ethically.