AI Innovations: What’s Next for Deep Learning Platforms
Exploring the Future of Deep Learning Platforms
The world of artificial intelligence is evolving at an unprecedented pace, with deep learning platforms at the forefront of this transformation. As businesses and researchers continue to harness the power of AI, the next wave of innovations in deep learning promises to revolutionize various industries. But what exactly lies ahead for these platforms?

Advancements in Neural Architecture
One of the most exciting developments in deep learning is the innovation in neural architecture. New architectures like transformers and graph neural networks are enabling more complex and efficient models. These advancements not only increase computational efficiency but also enhance the ability to understand and process unstructured data.
Transformers, in particular, have gained significant attention due to their applicability in natural language processing tasks, outperforming traditional models. As these architectures evolve, we can expect even more sophisticated applications across different domains.
Integration with Edge Computing
Another significant trend is the integration of deep learning with edge computing. This combination allows AI models to operate closer to data sources, such as IoT devices, reducing latency and improving real-time decision-making capabilities. This shift is crucial for industries that require instantaneous processing, like autonomous vehicles and smart cities.
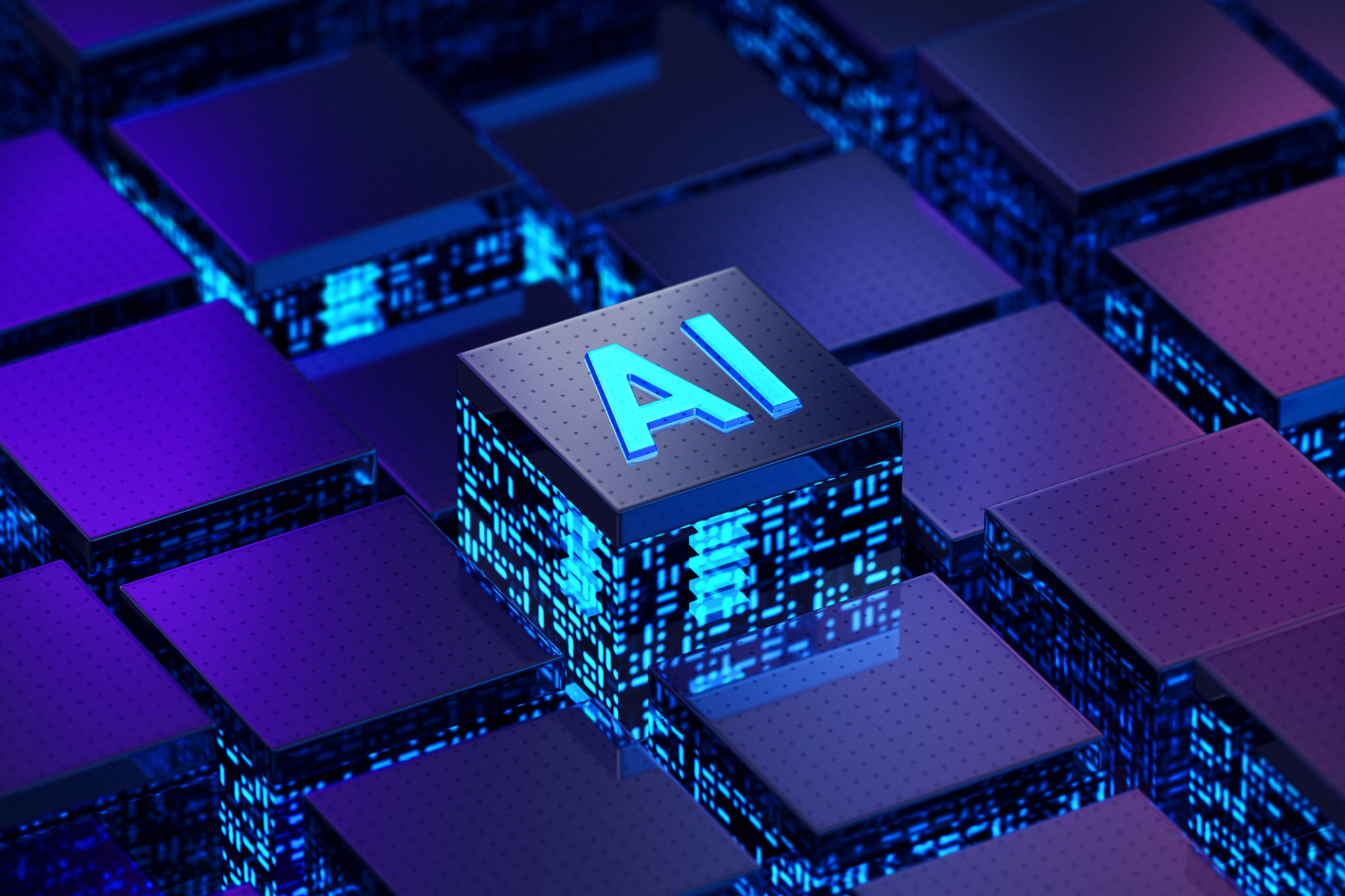
By leveraging edge computing, deep learning platforms can overcome bandwidth limitations and security concerns associated with cloud-based solutions. This integration is set to democratize AI deployment, making it accessible for smaller businesses and remote locations.
Ethical AI and Explainability
As AI systems become more prevalent, there is a growing need for ethical considerations and explainability in deep learning models. Ensuring that AI behaves responsibly and transparently is critical for gaining public trust and ensuring fair outcomes. Innovations in this area focus on developing models that can provide clear explanations for their decisions.
- Improving transparency in AI decision-making
- Ensuring fairness and avoiding biases
- Creating robust ethical guidelines for AI use
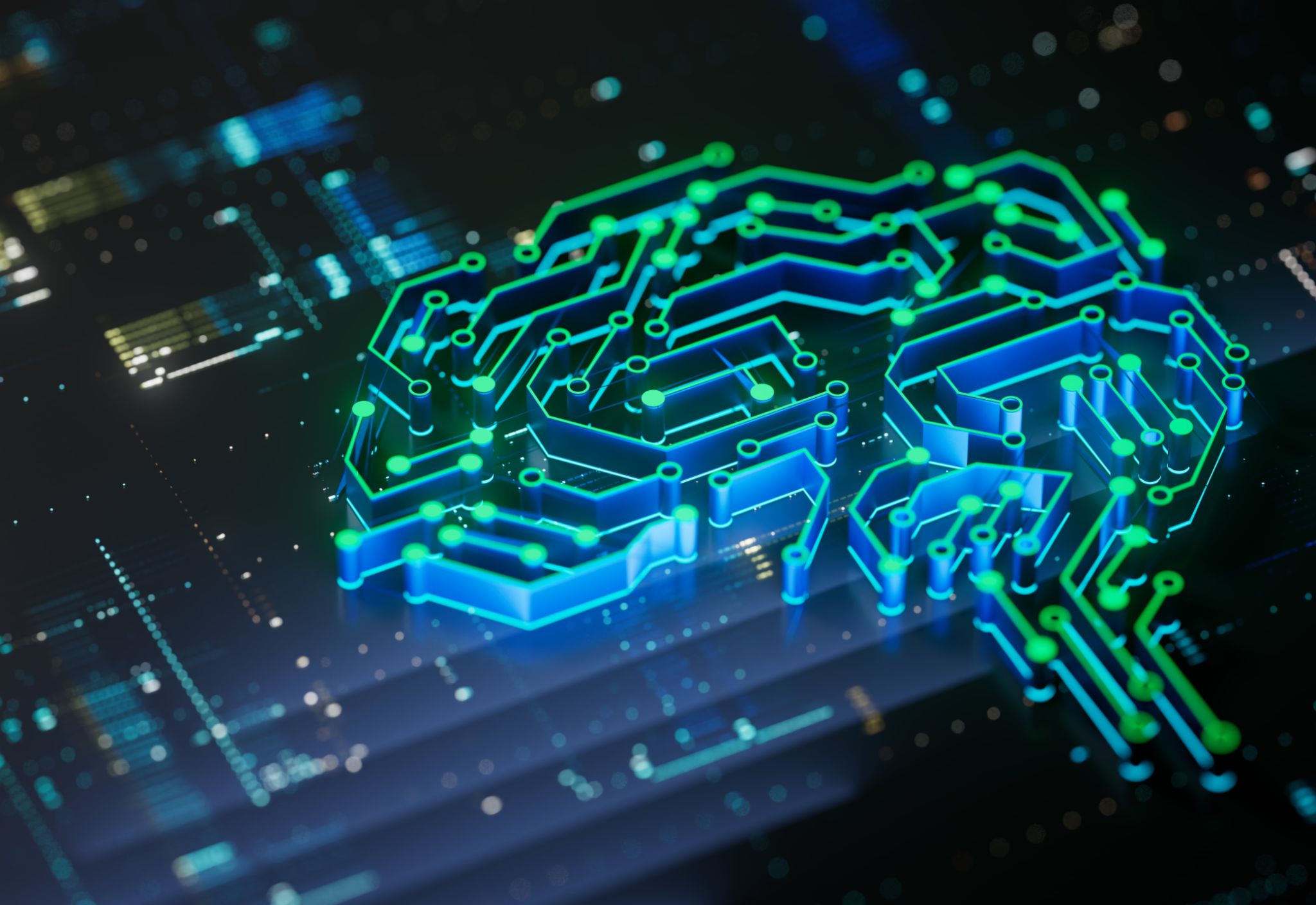
Automated Machine Learning (AutoML)
The rise of automated machine learning (AutoML) is another key factor shaping the future of deep learning platforms. AutoML simplifies the process of model selection and hyperparameter tuning, allowing non-experts to build effective models without extensive knowledge of AI. This democratization of AI tools empowers a broader audience to leverage deep learning for various applications.
Moreover, AutoML continues to evolve, offering improved user interfaces and more intuitive workflows. The ability to automate complex processes will likely lead to increased innovation across industries as more businesses can utilize these technologies effectively.
The Role of Quantum Computing
Quantum computing is poised to play a transformative role in the future of deep learning platforms. Although still in its infancy, quantum computing holds the potential to solve problems that are currently beyond the reach of classical computers. This capability could lead to breakthroughs in training times and model accuracy, especially for large-scale datasets.
While there are challenges to overcome, such as error rates and hardware limitations, ongoing research is paving the way for quantum-enhanced deep learning solutions. This intersection promises to redefine computational boundaries in AI.